Michel-Marie Maudet, Managing Director and co-founder of LINAGORA, Bastien Masse, General Delegate of the ClassCode Association, and Olivier Gouvert, Research Engineer at LINAGORA, were on hand for the event!
It was a moment eagerly awaited by members of the OpenLLM-France community, AI and Open Source enthusiasts, and all those curious to find out more about progress on LUCIE:the very first truly Open Source AI model, with 100% transparent training data.
More thana hundred people logged on to the webinar, demonstrating the real interest and relevance of this project for today's digital world.
Ambitions of the OpenLLM-France community
The LUCIE model is being developed by theOpenLLM-France initiative, launched in summer 2023 to promote sovereign open language models (LLMs). This initiative brings together a community of more than800 public and private players from French-speaking countries and Europe, including researchers, start-ups and businesses. The aim is to create digital commons for generative AI, by developing a language model accessible to all.
The project has also responded to a call from the French government to support the creation of sovereign language models, geared towards use cases such aseducation. Partners include theClass'code Association, a player in the education sector, as well as academic institutions and ministries.
The model being developed by OpenLLM-France is designed to be more open and ethical. Unlike proprietary models such as ChatGPT, this model will be entirely open source (under the APACHE V2 licence) and the training data will also be available under a non-commercial Creative Commons licence, guaranteeing transparency and free access.
The technical objective is to produce a model that is lightweight, energy-efficient and easy to deploy, to enable wider adoption in education, despite budgetary constraints.
Michel-Marie MAUDET, Managing Director of LINAGORA and co-initiator of the project, explains the project's ambition:
" Our aim is to develop a language model [...] that is simple and compact, that we can use quite simply without relying on major infrastructures that we don't control."
In short, OpenLLM-France aims to create an accessible and independent language model, adapted to specific needs, based on close collaboration between public and private players.
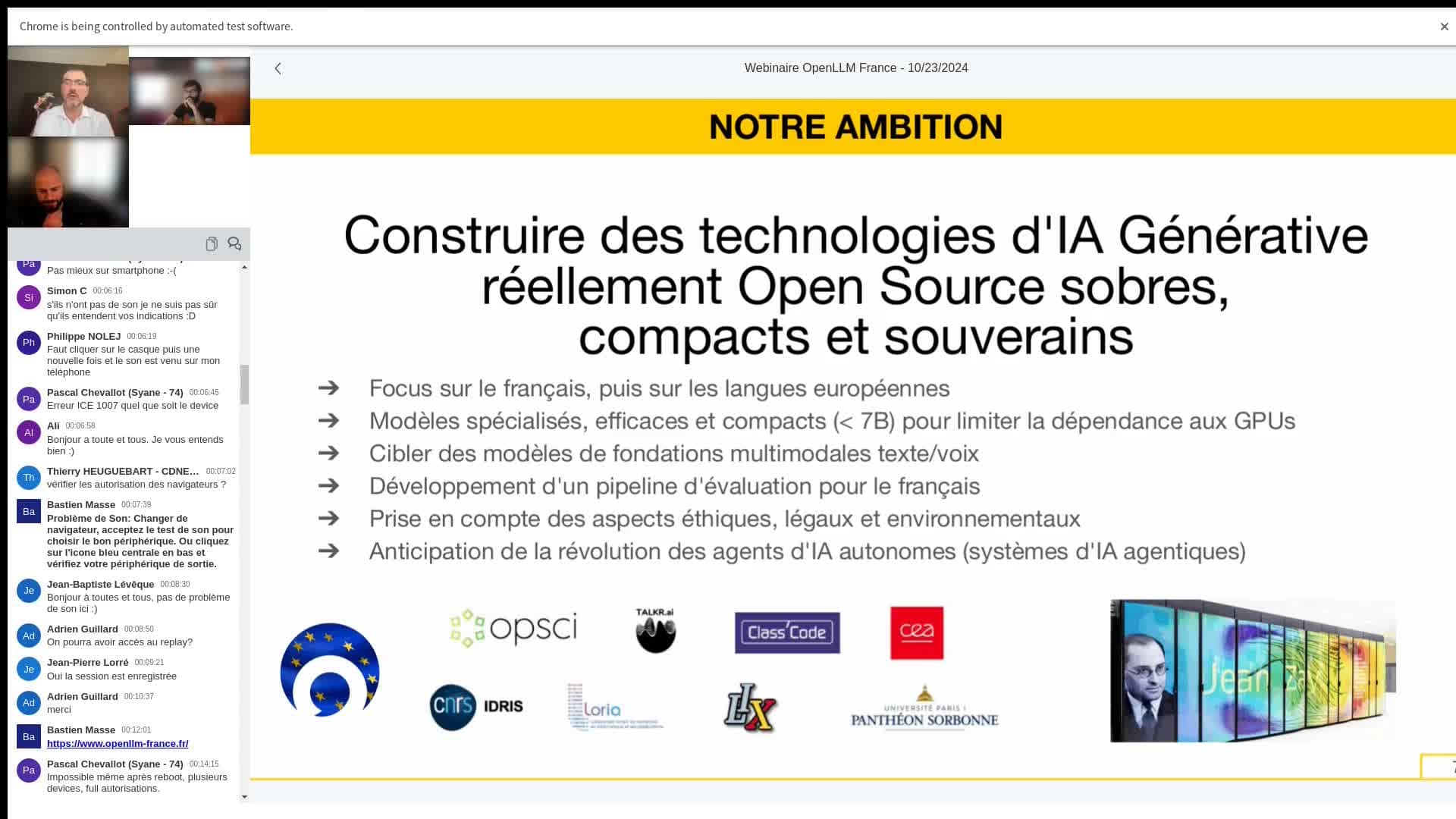
LINAGORA joins forces with Classcode under the OpenLLM-France banner
Class'code is an initiative set up in 2015 to train teachers in digital literacy and programming, using free educational resources. The association has already produced a number of e-books and a free MOOC in AI. It has also taken part in European projects, such as AI4O, to study the impact of AI in education.
Class'code works with the GTNUM Génial, a working group supported by the Direction Numérique pour l'Education and collaborating with the academies of Versailles, Nantes and Marseille-Aix. The aim is to understand how teachers and students use generative AI, and toidentify the challenges of evaluation, data protection and pedagogical approaches.
In this way, the OpenLLM-France initiative and Class'code are responding to the urgent need foroperational and ethical tools to exploit AI in education, with an open LLM and support in the field to train and equip teachers.
Bastien MASSE, Managing Director of Class'Code, explains
" Our aim was to bring together people from research, universities and other players to produce high-quality resources."
LUCIE, the Open Source AI model dedicated to Education
As with many sectors, education faces a number of challenges when it comes to integrating AI into the learning experience of students and teachers, particularly with regard to the need toacquire reliable,data-friendly and accessibleeducational tools. There are currently a multitude of solutions available, but few are adapted to the requirements of education (stability, compatibility with data protection, documentation, etc.).v
Lucie aims to meet these needs by offering a good alternative, independent of the major commercial players. This model has been developed to be stable and customisable, making it a suitable tool for EdTechs, teachers, researchers and students. In particular, it would enable applications such as RAG (Research Augmented by AI) for teachers, using reliable references to limit errors and bias. Teachers could thenintegrate their own content in a secure and controlled way.
A small version of the model (around 1 billion parameters) will also be developed later to enable the model to be used locally on low-power computers. This also made it possible to port the model to Raspberry or mini-computer type devices for secure use without the need for a network or exchange of server information.
The LUCIE model stands out for its simplicity, compactness and compliance with IACT, the European regulation for trusted AI. Designed to be entirely Open Source, Lucie is free to use, even for commercial purposes, and all the elements (datasets, code) are accessible, providing total transparency.
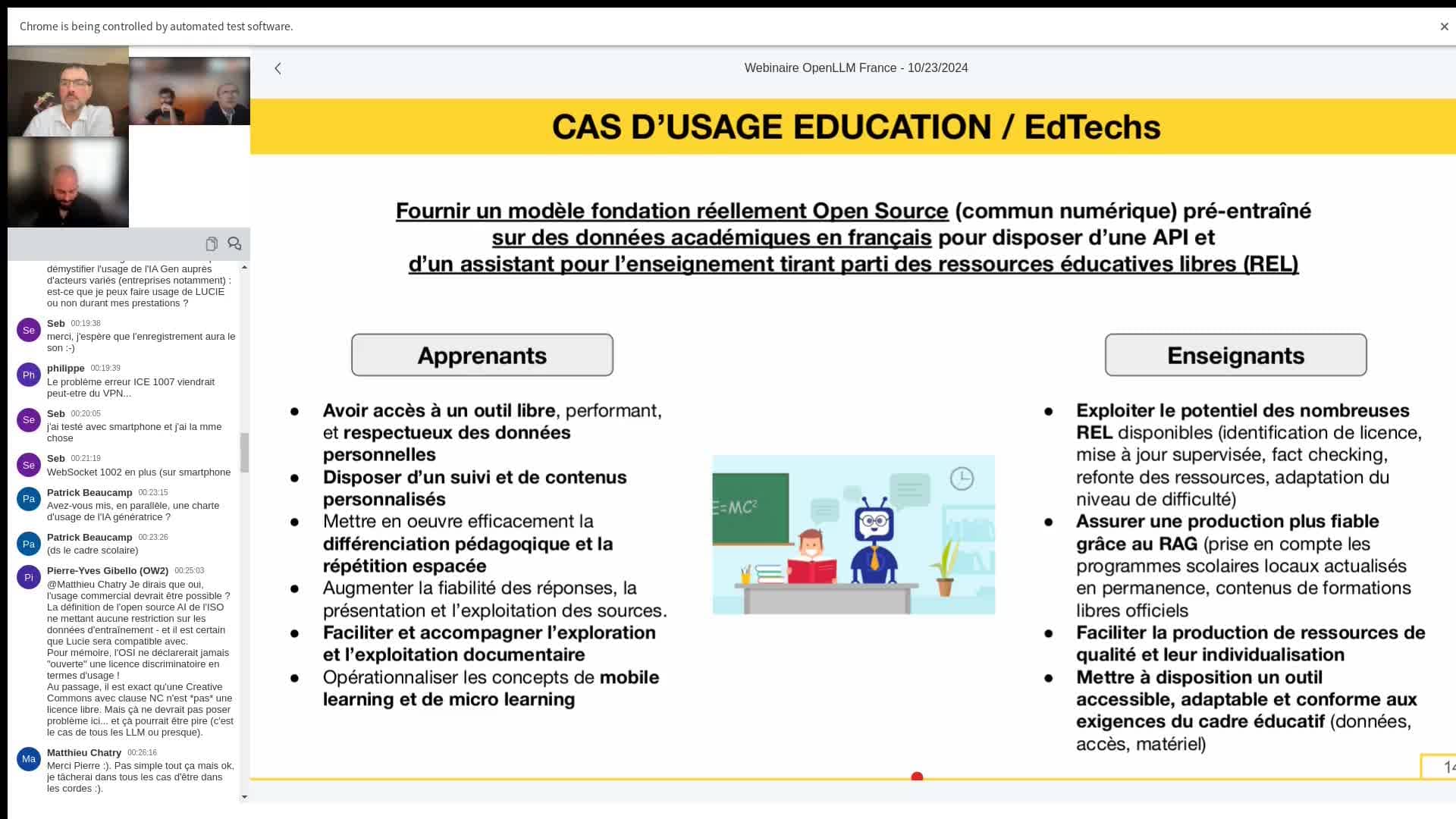
LUCIE's training
The training of this model began in August on CNRS infrastructure: the Jean Zay supercomputer at GENCI, with the ambition of processing 3,000 billion tokens to provide it with a vast knowledge base. This will require the use of 512 H100 GPUs in parallel and the collection of a massive data set.
" Without divulging too many figures, we are talking about 3,000 billion tokens, very large volumes that we will be making available as part of this process.
Michel-Marie MAUDET
- Composition of the data : To obtain a high-performance model, Lucie is trained on a mix of languages and data types: English (33% ), French (32.4%), computer code and mathematics (15%) because they improve the model's reasoning abilities. Other languages such asGerman, Spanish and Italian make up the remaining 20%. In this way, each type of data is weighted differently to prioritise quality (e.g. Wikipedia data is viewed several times) and diversify the model's language skills.
- Data filtering : To ensure optimum quality, strict filtering is applied. Data is selected according to quality criteria to avoid redundant, erroneous or offensive information. For example, URLs from sites deemed offensive or irrelevant are excluded, and content with banned words is filtered. A ‘near deduplication’ process is also used to eliminate repetition and guarantee data uniqueness.
Data scheduling and training parallelism : Lucie's training runs using GPUs in parallel and applying a ‘3D’ parallelism technique to optimise the process. Older data is processed at the beginning of the training run, allowing the model to be refocused on up-to-date information at the end. This choice helps Lucie to keep her knowledge up to date.
Olivier GOUVERT, Research Engineer at LINAGORA, explains:" We find it easier to accept that he's forgetting things about old data "
- Context window expansion: Lucie is currently in the process of expanding its context window from 4,096 to 128,000 tokens, enabling it to better handle long texts without breaks. This capability is crucial for applications that require a great deal of contextualisation, such as RAG systems. For example, in a question-and-answer application for a company, this would allow the model to consult an entire company document, enriching the relevance of its answers.
Performance evaluation and comparison: Lucie is compared with other models such as Pythia, Croissant LLM and Falcon, on French and English benchmarks. Its performance progresses logarithmically, and the results show promising forecasts. On the left of the graph, the English benchmarks show the stages of Lucie's progress compared with predominantly English-speaking models, while the French benchmarks highlight her increased proficiency in that language.
Olivier GOUVERT emphasises:" We can see that our tokenizer is adapted for these 5 languages, which we have chosen to represent the diversity of European languages, as well as for the code."
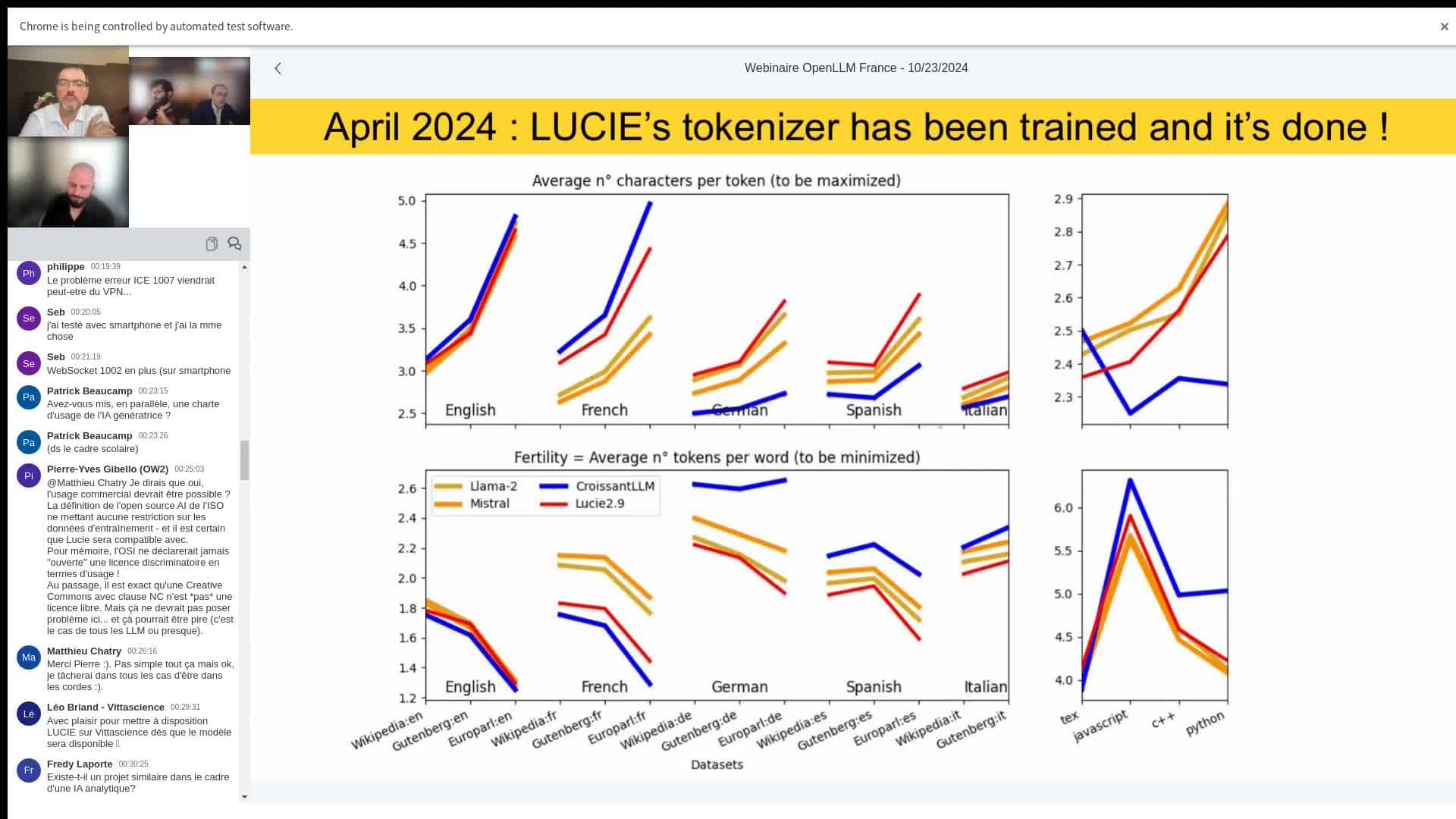
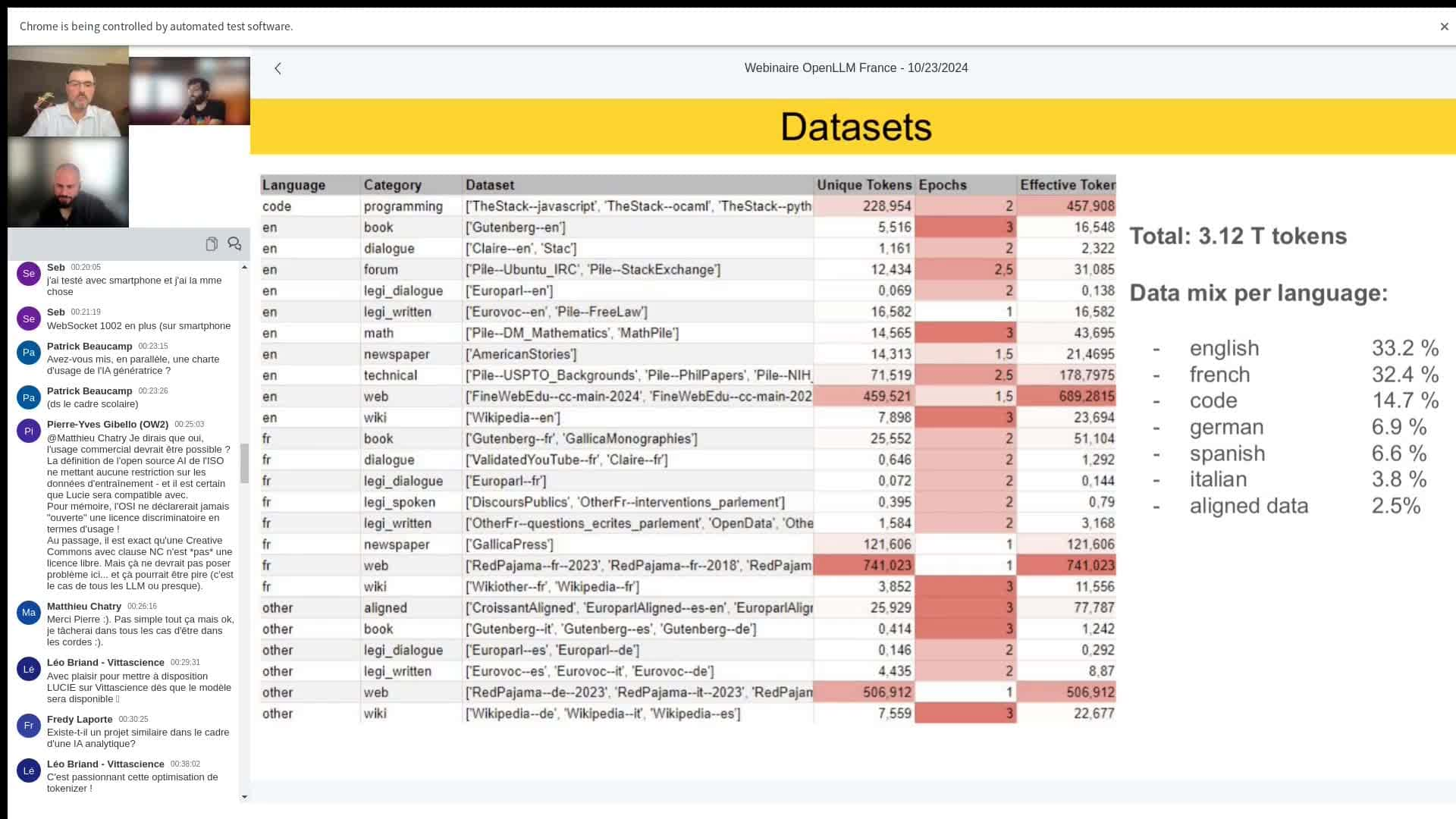
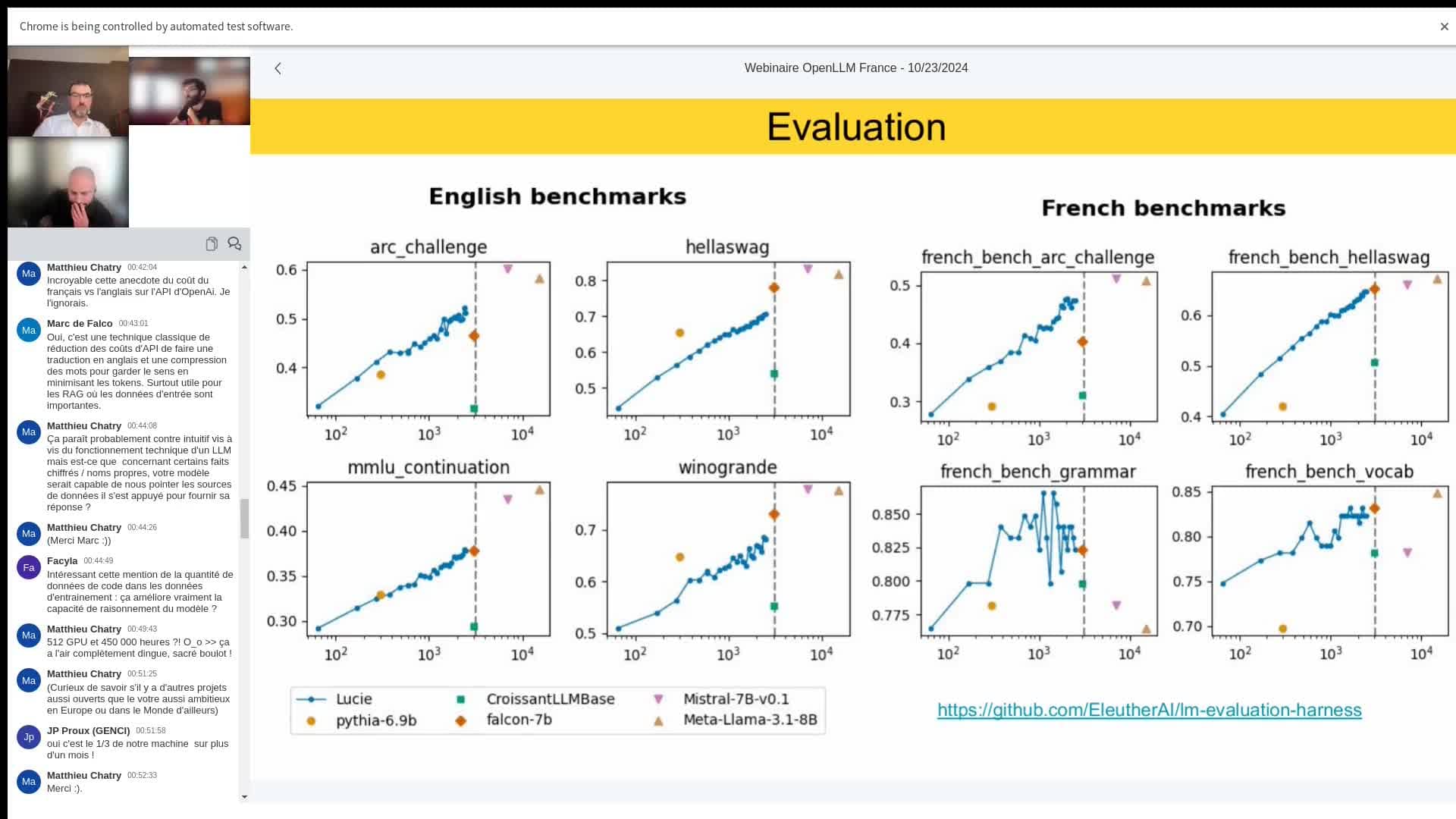
6. Instruction and alignment phase: In order to improve her responses and align them with human expectations, Lucie goes through a two-stage instruction phase:
- Fine-tuning: This first stage involves adjusting the model on question-and-answer data, which is closer to human discussions.
For example, specific datasets are created with simple questions such as ‘ What is the capital of France?’, and precise answers are provided. This process teaches Lucie to give a direct answer, such as ‘ Paris “,” explains Olivier.
- Reinforcement learning: In this second stage, Lucie generates several answers for the same question, and these are evaluated. Human preferences are then integrated to refine her future answers.
Currently, the Lucie model is carefully trained with a combination of multilingual data, meticulous filtering and advanced training strategies. This ensures that the final model is both diverse and efficient for complex comprehension and text generation tasks.
What happens next ...
Michel-Marie MAUDET outlines the next steps:
" We're really planning this for early December. We'll be publishing the model, the data-sets, the source code and all the sauce we've used to train the model."
The OpenLLM-France teams are currently working on finalising and pre-building Lucie, with the aim of producing a final model by the end of November. The public release is scheduled for early December, with the publication of all the resources, such as the source code, datasets and training methods, in collaboration with Hugging Face.
Lucie will be available on this platform, along with a free API for testing. Users will be able totest or download the model for use with local tools such as Ollama and LM Studio.
Access will also be possible via the Vittascience platform , an Edtech partner in the project, which already offers scientific mediation activities on AI. This will enable students and teachers to access the platform without having to log on.
LINAGORA is also planning a SaaS platform with a subscription model for using Lucie, to cover infrastructure costs, mainly due to the GPUs required.
A slimmed-down version of the 1 billion-parameter model is also in the pipeline. This is aimed at use on mobile devices, including telephones, to make access to large language models possible in a local context.
All the information, including the source code, will be available on the community's GitHub: https://github.com/bentoml/OpenLLM